This website uses cookies so that we can provide you with the best user experience possible. Cookie information is stored in your browser and performs functions such as recognizing you when you return to our website and helping our team to understand which sections of the website you find most interesting and useful.
Machine Learning and Natural Language Processing Case Studies
The digitalization of society has led to vast amounts of new data that also come in new types. From transactional data that capture events in the field, to electronic health records to geolocation from sensors, images, or text, we have developed methods and tools that make sense of this wealth of information. Machine learning (ML), with its ability to extract regular patterns from all types of data, opens new possibilities for our researchers looking to augment traditional research techniques.
In collaboration with subject matter experts and methodologists, our data scientists develop applications for natural language processing (NLP) using both traditional and cutting-edge deep learning models in a variety of tasks––from the identification of key information in interviewer comments in traditional surveys to the classification of clinical notes in electronic health records.
We embed ML models in data collection projects to identify the most cost-effective strategy to gain cooperation from survey respondents or to detect potential interview falsification. Using these new methods, we have built new tools to extract insights from images, videos, or audio files to improve the efficiency of data collection, evaluation, and analysis.
Drug Abuse Warning Network (DAWN)
The Substance Abuse and Mental Health Services Administration’s (SAMHSA’s) DAWN study collects data in 50 hospitals across the United States. The goals are to (1) identify new and emerging drugs and use patterns, (2) be an early warning system for drug-related events, and (3) produce immediately available data. Our challenge is to provide continuous review of emergency department (ED) records to identify key data elements in drug- and alcohol-related visits.
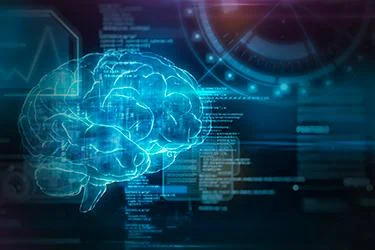
To ensure rigorous data quality and keep costs low, Westat developed ML models to review and route DAWN data to expert reviewers who must decide whether a drug caused or contributed to a person’s ED visit. The models Westat developed assign a probability score indicating whether the ED visit is likely to be in scope for DAWN and the likely category of the visit. These models are retrained periodically to increase their efficiency. The result is that DAWN data are of very high quality without relying on human review of each case.
National Diabetes Surveillance
As part of our work for the CDC’s national diabetes surveillance strategy, Westat developed and fielded a telephone survey of patients with diabetes in a large health system and acquired matching EHR data for the survey sample. By linking these 2 sources of data, Westat was able to validate survey-based and EHR-based algorithms to determine patients’ type of diabetes against a “gold standard” diagnosis achieved by manual review of patient charts. Using a supervised ML model, we were able to develop a conditional inference tree that classified each adult patient into type 1, type 2, or other diabetes type with very high accuracy.
Medical Expenditure Panel Survey (MEPS)
During data collection, field interviewers often append electronic notes or “comments” to a case in open text fields to request updates to case-level data. These comments might contain actionable information that alerts data technicians to unusual responses or circumstances that can affect data quality. Trends in topics or content of the comments may provide valuable insights on imperfect question design, training gaps, or bias from an interviewer.
At the same time comments are often superfluous or do not contain enough detail to be actionable, and processing comments is time consuming. The ability to reliably assess these comments and apply standardized data editing procedures quickly is key to improving data quality and increasing efficiency.
Westat developed a novel application of ML technologies to assist in the evaluation of these comments. Using thousands of comments from MEPS, we built features that were fed to a ML model to predict a grouping category for each comment. The model achieved high accuracy and was incorporated into a production tool for editing. A qualitative evaluation of the tool also provided encouraging results. This application of ML created an increase in processing efficiency while maintaining exacting standards for data quality.